Pacers can close the series in Cleveland, Donovan Mitchell is questionable, and there is an average of 48.5 fouls per game in this series. These are just a few ingredients for a disastrous same-game parlay for game 5 of the Cavaliers-Pacers series.
Perilous Parlay Is Redundant
If you are betting a parlay a day, then words from a rando probably won’t change that. However, if you are after sustainability and consistency (or something close to it), then gee golly wiz, parlays aren’t it. Though betting in general probably isn’t it. End of speech.
Now, because I like doing parlays every now and then for fun, let’s look for a convoluted way to lose some dollars.
THe Feels Parlay
To add to the fun, I am going to do a parlay based on “the feels” and a parlay based on the data to see which comes closest to hitting. Not quite a scientific method but fun nonetheless. At least it seemed like fun when I came up with the idea. Now it just seems like a waste of time. And a waste of doughnut money.
For the “Feels Parlay”, I will tell you that is based on my unrivaled instincts, hours upon hours of watching game footage, and match-ups that I find favorable. In reality, it will be filled with bias, assumptions, and picks based on increasing the parlay odds to the sky.
So, here is my parlay based on my unrivaled instincts, hours upon hours of watching game footage, and match-ups that I find favorable.
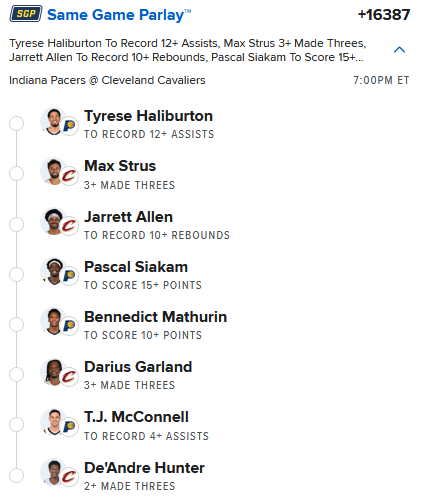
The bonus of going off “the feels” is that this only took a minute to enter. However, the odds when I clicked the button to submit the bet (over +21000) dropped to +16387 in a matter of seconds. I expect the drop to continue.
The Data Parlay
Now I will lose track of time reviewing data modeling results, game logs, and any other resource that I can find to “the feels” out of the parlay.
After running a data model on the projected starters and a few bench players, but before looking at other data points or betting lines, here are some of the players and numbers that stand out.
- Tyrese Haliburton: 20 points, 9 assists and 2 3-pointers
- Andrew Nembhard: 9 points
- Pascal Siakam: 7 rebounds
- Bennedict Mathurin: 12 points, 2 rebounds
- T.J. McConnell: 2 rebounds
- Darius Garland: 8 assists
- Donovan Mitchell: 30 points, 4 assists
- Max Strus: 11 points, 4 assists
- Evan Mobley: 15 points
- Jarrett Allen: 11 points
- De’Andre Hunter: 14 points
- Ty Jerome: 11 points, 2 rebounds, 3 assists
Outside of the fact that I like looking at multiple data points before running with the predictions, there are other pieces of information to keep in mind before I just run with the numbers. For example, Donovan Mitchell is a game-time decision. I think that is a known unknown. Also, Mathurin and Hunter are using their play time to play tag which is a known known. Though I don’t understand why so maybe that is known unknown.
Next, I will compare predicted stats to game logs, hit rates, etc. Even though I have a value in the model that gives me a confidence score (for the lack of a better term), I want to check the numbers to see how “reasonable” they are or how likely they are to happen.
Below is the “Data Parlay”.
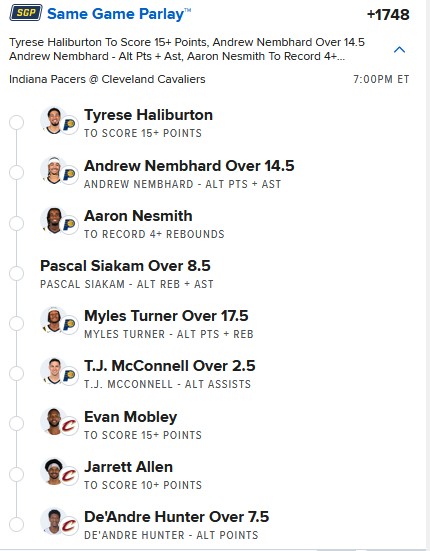
Now, I try to forget that I spent so much time on this and look for a straight bet to get my meager Tuesday win.